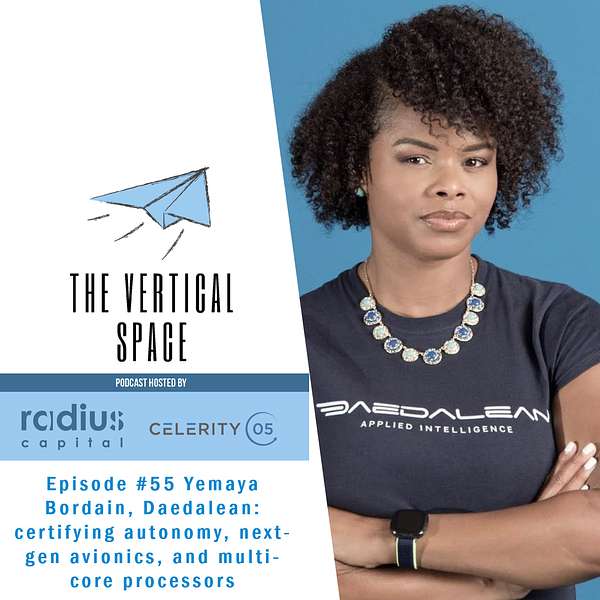
The Vertical Space
The Vertical Space is a podcast at the intersection of technology and flight, featuring deep dives with innovators, early adopters, and industry leaders.
We talk about the radical impact that technology is creating as it disrupts flight, enabling new ways to access the vertical space to improve our lives - from small drones to large aircraft. Our guests are operators and innovators across the value chain: airframers, technologists, data and service providers, as well as end users.
The Vertical Space
#55 Yemaya Bordain, Daedalean: certifying autonomy, next-gen avionics, and multi-core processors
Check out an in-depth and fast-moving discussion of certifying autonomy, next-gen avionics, and multi-core processors with Dr. Yemaya Bordain, President of the Americas for Daedalean.
Right out of the gate Yemaya challenges much of today’s established thinking on UAM: Listen to what she says is an area where very few in the industry agree with her on – and where she questions the near-term economic viability of the UAM model. And that she believes level 4 autonomy is needed for UAM economic viability – and listen to later in the podcast on when she believes level 4 autonomy may come about. Those investing in UAM should pay close attention here!
We discuss the history of automation, the Why of automation, on a segment by segment basis, what has and will drive the need, and how does it scale, followed closely by a detailed discussion of the levels of automation, and the value of each– and why. And how safety improvements are the biggest near-term opportunities in this market.
Listen to Yemaya’s first discussion with operators on autonomy – particularly commercial operators. The next part of the discussion is a detailed discussion on the future of avionics, starting with a discussion of Yemaya’s white paper.
Listen how to compute massive amounts of data to meet the size, weight, and power constraints of gravity. We enter into a detailed discussion on why AI needs multi-core processors and the difficulty of certifying MCPs and listen to Yemaya’s response to Luka’s question on whether or not there can be a generalized approach to certifying MCPs and how Yemaya thinks that autonomy and certification of AI and MCPs will change the existing value chain in avionics.
She also discusses at what levels of automation where Daedalean will be generating revenue, and in what markets, including AAM, over the next 10+ years.
when I started at, Daedalean, I was so focused on how cool the technology was that I just assumed that he got it when I said automated or autonomous. Why weren't you so excited? And he said, well, one, I don't think that automation or autonomy is going to happen for next decade. that makes you not very useful to me, right now in, in what you're providing, if you're leading with that. but secondly, he said, Yemaya, we are not, a risk environment. We, are margin constrained and. It was like, Oh, right and so what I realized is that we need to solve the problem. I was trying to solve a technological problem, but we're really supposed to be solving an operational problem. And that operational problem is an economic viability issue.
Jim:hey everyone. Welcome back to The Vertical Space and our discussion with Dr. Yemaya Bordain as president of the Americas of Daedalean. From right out of the gate, Yemaya challenges, much of today's established thinking. Listen to what she says is an area where very few in the industry agree with her on. And where she questions the near term economic viability of the UAM model. And that she believes level four autonomy is needed for UAM economic viability. And listen to later in the podcast, when she believes level four, autonomy may come about. Those investing in UAM should pay close attention to Yemaya's comments. We first discuss the history of automation. The why of automation on a segment by segment basis. What has and what will drive the need and how does it scale? Followed closely by a detailed discussion on the levels of automation and the value of each. As well, as in what market the Daedalean is spending most of their time today and why. And how safety improvements are the biggest near term opportunities in this market. Listen to Yemaya's first discussion with operators on autonomy, particularly commercial operators. What's also interesting is the role or lack of a role for AI at the different levels of automation? Next is a detailed discussion on the future of avionics, starting with discussion of Yemaya's white paper. We enter into a detailed discussion on why AI needs multi-core processors and the difficulty of certifying MCPs. And listen to Yemaya's response to Luka's question on whether or not there can be a generalized approach to certifying MCPs and how Yemaya thinks that autonomy and certification of AI in MCPs will change the existing value chain in avionics. She also discusses the levels of automation where Daedalean will be generated revenue and what markets including AAM over the next 10 plus years. Her summary at the end of the discussion is inspiring. Yemaya, thank you for joining us and to our listeners, enjoy this terrific discussion with Dr. Yemaya Bordain as you innovate and The Vertical Space. Dr. Yemaya Yemaya Bordain joined to Daedalean in October, 2022, as president of the Americas to lead innovation, flight testing, and partnerships. Previously, she spent seven years at Intel developing industry, leading to advances in partnerships with top global OEMs, including Lockheed Martin corporation, Collins Aerospace, Indra systems, and Mercury Systems. She earned her BS in electrical engineering and MS in Computer Science at Clark Atlanta at University and a PhD in Electrical and Computer Engineering at the University of Illinois, Urbana Champaign.
Luka:Yemaya, welcome to The Vertical Space. It's a real pleasure to have you on.
Yemaya:Well, thank you so much for having me. I'm so excited to be here today.
Luka:We are, too. We are, too. So let's start with the one thing that very few in the industry agree with you on.
Yemaya:Lately, I've been going to and even speaking at some of these Urban Air Mobility, conferences and I think that there's a business case, problem that, Urban Air Mobility has particularly for people carrying, operating cost, the cost of total ownership, the cost of the airframes, less range, slower, and carrying people, especially when they need to carry luggage, I just think that there, there should be some, analyses that is less optimistic than some of them that I've seen recently and, more feasible and truly take into account, how the airspace is operated today.
Luka:Right. so the need for high levels of autonomy to scale is what you'd like to underline in this viewpoint, right?
Yemaya:Yeah, the economic viability in, our minds, it doesn't happen until one is able to scale and automation, autonomy, these will address the operating cost significantly and enable that scale. And so we believe that we don't see economic viability until at least what we call like a level four, autonomy.
Luka:Interesting. let's set the stage, understanding that aviation has a rich history in automation from a hundred years plus. And so describe to us the evolution of automation in aviation from its very early days to today and how we think about autonomy.
Yemaya:Oh man, great question. I guess when we think about it in terms of that, automation and autonomy could potentially be its own scale, in relative scale versus absolute scale. I'm certain that, Sperry's gyroscope, seemed like a huge, device that drove automation. and it came only, what, six years after the Wright Brothers, flew, right? some other huge advancements included Fly by Wire, enabling, pilots. to fly the aircraft through a computer. that, that was huge, right? And in fact, one of my favorite technical papers that exists is, the, fly by wire system architecture that was published on the A320. It's, it honestly, it's actually what taught me how, important flight safety is and how people design safe systems. And then, finally today, autonomy looks like everything, being Digital, all pilot tasks moving toward being taken over by, ultimately silicon plus algorithms.
Luka:Yemaya, historically, the introduction of, automation in flight. as I describe it, was initially because of the expansion of the envelope of the aircraft. aircraft became, faster, they flew higher, they flew farther. So there was a need to alleviate the pilot mechanically from, the stick and rudder skills. And it was much more difficult with these higher dynamic loads to move the control surfaces and therefore increasing level of automation. And then, I guess another phase could be the use of automation to reduce the cognitive load of the pilot to then, paint a better situational awareness picture or just operate more efficiently and safely in the increasingly, busy airspaces and complex scenarios. And so what about now? What is this? Is this a scale? the next frontier that introduces this new wave of automation?
Peter:Luka, you mean in terms of the motivation for, why are we pursuing this?
Luka:Yeah. this arc of introducing various degrees and kinds of automation and what is really the motivation.
Yemaya:Yeah, I think you're right on with scale, accessibility, cost. In today's, general aviation market, it's, safety enhancements. general aviation has so much headroom for safety and automation can enable, GA to enjoy the safety levels that are available to the commercial market. I do believe that it's driven by cost, it's driven by scale, it's driven by, people wanting more accessibility. It's driven by our world, being more connected and requiring, being able to, engage a lot faster, right? There used to be email and, You could expect that someone would respond to your email within two days or then within a day. Now you just text people, right? So I think a lot of the driving forces behind it is really, along the lines of the trends of society today, mostly driven by scale and cost.
Luka:Peter, since you asked, what jumps to mind to you when we introduce this arc and this motivation?
Peter:Well, I think it's really interesting to look across the segments of aviation and, okay, number one, you look at the opportunity to automate, and then number two, you look at, well, what differential does it deliver and let's say in commercial aviation, it is, It's exceptionally safe today, it has a level of automation but it's not the type of automation that we're looking ahead to, nor is it, a level of automation that you would describe as autonomous behavior. but in that segment, there is, a lot of resistance to, bringing in high levels of autonomous flight, reducing cockpit crew from two to one. there are a lot of motivations that people are pursuing it, but there's a lot of resistance to doing that. in other parts of aviation, you have a different landscape. You do have a safety opportunity, but you might have, a different customer profile. Where I think it's really interesting is some segments of aviation, UAS, they're physically so small that putting a human on board to operate it is just, fundamentally not an option. This is a 40 pound air vehicle. And so in those segments, you have an incredibly high motivation to, bring the level of automation up to whatever is required for the mission, call it autonomous flight in some cases, if you want, because that's what makes the, mission possible. and in some of these segments, as you highlight, making that mission possible is world changing. It will change our perception of how we think about moving things around the world, how we think about the time and space around us. given all these different, segments of aviation, they share some technological similarities, they might be operating a different scale, it might be a different mission profile, but there's a lot of commonality in the technology that pulls this off, that allows us to achieve this vision and looking at it through that lens is what... has me so interested in this question of motivation because like everybody, I just took it for granted that of course we want autonomous flight. It's a new technological horizon. Let's go and build it. But then in conversations with people in industry, it struck me like, wait, let's take a step back and think about what is the real motivation and which segment of aviation is the path of least resistance to build up the technology, to build up the operational experience, that kind of organizational muscle, to then really realize the vision. And maybe it propagates from there, but where do you start?
Yemaya:so I, to address What you stated, it's such a good point. by training, I'm an engineer and I absolutely positively nerd out on new technology, and I find that much of the industry does that as well. And so your point of course we want automation. Of course we want autonomy. I initially geeked out about that as an engineer and just, this is so cool. We totally should do this. And it was after the fact that I challenged myself and my team to dig into why do you want autonomy? What does automation and autonomy deliver? And that's when we were able to, realize or, reveal that we want enhanced safety. We think that this can reduce labor cost. we think that this can enable the optimization of crew, it can streamline operations, potentially even, lead to simpler maintenance, fuel and energy consumption. We just, we realized that we wanted to focus more on the why of automation and autonomy, and of course, be pioneers of how we get there as well.
Jim:Yamaya, it's interesting. you proud yourself on being a problem solver. I've read a lot about you and I've seen you in interviews and we're talking about the foundation of why autonomy, but the reason you say some people may not agree with you is that you're saying that the advanced air mobility passenger carrying world won't be viable without what you're claiming to be fully autonomous, which is, four out of the five stages. So you're saying that the very business proposition of AAM won't be viable without autonomy. So that's a pretty darn good reason. A lot of us agree with you on that. And so if we need a motivation... For autonomy and advanced air mobility, this is one of them, because even the public companies are saying that we can be profitable, but for them to be able to meet their financial projections, some level of autonomy, you call it fully automated automation, as
Yemaya:automation. Yeah,
Jim:You're saying that has to be achieved. So what a motivation.
Yemaya:Absolutely. Absolutely. And I think that you can only get there when you sit down and start talking to operators. and in particular, you start talking to today's civil airspace, those operators. I remember having, meetings with a very large operator and, I was so focused on how cool the technology was. And, this is when I started at, Daedalean, I was so focused on how cool the technology was that I just assumed that he got it when I said automated or autonomous. And I didn't get the same kind of excitement from him that I expected. And I took him to the side and I said, Hey, can you give me, very direct feedback? Why weren't you so excited? And he said, well, one, I don't think that automation or autonomy is going to happen for next decade. that makes you not very useful to me, right now in, in what you're providing, if you're leading with that. but secondly, he said, Yemaya, we are not, a risk environment. We, are margin constrained and. It was like, Oh, right and so what I realized is that we need to solve the problem. We're problem solvers, each one of us, even, the engineers. I was trying to solve a technological problem, but we're really supposed to be solving an operational problem. And that operational problem is an economic viability issue.
Luka:Right. we had, several guests in the course of the podcast series where we discussed autonomy and it's always interesting to hear their thoughts on the reasons why autonomy, we talked a little bit about this now over the last several minutes, but I think it's worthwhile hovering a little bit more and going to a bit more detail, perhaps on a segment by segment basis, commercial, airline operations, part 121 operations general aviation, UAS, all of the other segments. And describe what are some of the common misconceptions in terms of the reasons for autonomy, versus what you think autonomy is helpful for, right? what's in the back of my mind is a conversation that we had with, Brian, Yutko from, Wisk, and on that topic, he mentioned that, a common misconception is that people tend to drive their economics discussion around autonomy in terms of the removal of the cost of the pilot in the operation, whereas he says, no, that's totally not the point. The point is in a better management of an asset, from a network perspective and being able to reposition those assets and separate the human operator from the actual vehicle and how that opens up the, operational freedom and the network design freedom, which totally makes sense and similarly, there are. misconceptions around safety where people jump to the conclusion that, removing the pilot will automatically result in safety. So give us your thoughts around this. It's a kind of long way of saying, let's go a layer deeper into why autonomy.
Yemaya:Yeah, I, absolutely positively agree with Brian. We also are not driving toward removal, or, nor do we think that in, in every, application, the removal of a pilot is the best thing. And in fact, when we deliver talks and talk about the levels of, autonomy, we've started looking at those in terms of Search and Rescue, there's, Offshore Oil, Transport. There is, Law Enforcement and Surveillance. We, there's HEMS, there are many that we said, yeah, these could be along the spectrum. of, automation, but they're not appropriate for full automation and full autonomy. And, we define the difference between the two of those, as full automation the aircraft is, self separate, but there is a human in the loop. who is monitoring, the decisions that are being made and then for full autonomy, there's no human in the loop. This thing just runs, by itself uninterrupted and unmonitored. And, so for that reason, we think that it is a common misconception that saying that you want to, develop autonomous or automated systems means that you want a world where there are no pilots. it's quite the opposite. We want pilots to, be managing the decisions, the processes, that are reserved only for humans that's where we think that, it continues to be in, especially in very, dynamic and, environments that are changing quite a bit. And even in those, we talk about that a bit as well of when should you use AI versus not AI and where there are benefits. But, we want to augment pilots, we want to support pilots, we want to lower the pilot workload, we want to enable operators to scale, we want to address the cost by using, resources a lot more efficiently.
Luka:When
Peter:When you looked across, those different operations and CONOPS, on the one hand you look at the ultimate manifestation of autonomous flight and you say, okay, well, where does this really fit in? And maybe it's transoceanic cargo as you mentioned, did the stepping stones to get there also surface from all of those conversations where you see, hey, okay, here's the state of the art today. And if we push one step further, what is a sensible application for that where we could, deploy it, build up experience with it and have a marketable. solution out there. did the path to get there, surface from those conversations? And I'd love to get your thoughts on what that looks like, whether we really need AI or not in order to go down that path. what did that look like? what emerged from that
Yemaya:Yeah, absolutely. We looked at these different CONOPS. we approached it in a few different ways. We looked at the, segments, the market segments within aerospace and, not including defense. we don't, support defense applications as a company. and we looked at GA, we looked at commercial, we looked at even, UAS and we looked at UAM, it as its own kind of use cases or segment as well. because we do think that it in the way that it will, be developed, it'll be, a pretty unique, use case or a pretty unique segment. we looked at those and we segmented them in margin versus volume. We, also looked at, the, pilot tasks that can be automated, as you go along. So for instance, being able to detect obstacles or other, aircraft, in the airspace. and we looked at it in, in ConOps and what we were trying to get to, is where automation and autonomy could address, a segment that, is currently high revenue generating, even if it's not high margin, because the costs are so extreme, or the costs are just, they're prohibitive, put it that way. and we thought through some of those applications and then we dug more into those and what parts of that if we were to, automate certain tasks or automate, certain parts of, these operations, what, how could we get a bigger bang for our buck, in order to enable, these things to scale? We also, took some applications that we think probably would fall under kind of lower automation, say for instance, Search and Rescue, where, you know, the environments are really dynamic and the mission itself is dictating, say, the navigation, of the airframe, right? And in those cases, we thought that, okay, how can we support the pilot workload, the pilot that is, flying, but then there's the, operator that's managing the mission, right? That's basically telling the pilot, go here, go there. So we thought about that as well. And we said, look, we just don't think that there is a huge opportunity here, but there are definitely some specific tasks and areas where, we can assist the pilot. And so we thought through that as a spectrum and we went through tens of CONOPS in order to rank them along, different scales.
Jim:You have mentioned level four and level five automation. do you mind just mentioning the five just for our audience? Because it's cool how you refer to CONOPS and levels of automation, but if you could just, go from assistance to partial automation to highly automated, just briefly define them for our audience. And then as you refer to from level one to level five, they'll have a sense for you, what you mean by, for example, even where's the low hanging fruit for automation.
Yemaya:Yeah, absolutely. Level one, human has, full responsibility. And this is just for task assistance. So the AI, Pilot Not Flying or, AI Co Pilot. Sometimes we call it AI Co Pilot. Sometimes we call it Pilot Not Flying or Pilot Monitoring it provides just assistance to the pilot for, situational awareness. And the AI provides... situational intelligence. So this is the ability for the pilot to not only understand the current situation, but also to be able to predict the future and anticipate, any potential problems in the future. So what humans do today with semantic understanding is that we paint a picture and we're able to identify threats that could happen in one second, five seconds, 10 seconds. That's what the AI brings. And in this case, we are just providing the pilot with task assistance. and our first product, PilotEye is a great example of this. PilotEye is a traffic advisory system that uses, camera inputs and neural networks to draw a box around anything else that's in the airspace. And, what is the value proposition there? Well, when you're using cameras and neural networks, we identify non cooperative traffic. We identify birds and drones and paragliders and, hot air balloons, right? And the point is not necessarily to say this is exactly where it is, but the system, it provides that input to say, look up. There's something there, and we, we want your attention here because we see something there that is not, going to be identified, through a transponder.
Luka:And Yemaya, what segment of the market are you targeting with Pilot Eye?
Yemaya:Yeah, PilotEye is, GA.
Luka:that's interesting because as we were discussing earlier, GA is the segment where, safety is a concern more than for other segments of the market. But at the same time, it is a segment that doesn't really have a high willingness to pay for new technology. So how have you thought through the business case. How do you articulate value?
Yemaya:Yeah. Oh my gosh. That is such a great question because this is the, it's the ongoing question, right? And sometimes it's even, what divides your BD team from your engineering team? Because engineers want to do cool stuff. and engineers want to develop the best of the best and we have to remind our teams that, Hey, just to let you know, right, these things need to sell for 15K, 20K, right, 30K.
Luka:Right. And at the same time, the community is struggling to sell 500 transponders,
Yemaya:Right. Absolutely. this is where that analysis that I spoke of earlier, where we assess the market along ConOps, but also along, the high revenue generating, ConOps and applications because there is a bit more flexibility in those markets and then we drive toward there. And there are some applications where. I've had conversations with, operators and they've stated, yes, we won't pay, I'm making it up, a hundred K for this, but definitely the cost benefit justifies, us paying more for this because this is a real threat for operations and it could lead to loss of airframe, which in that case, then you're talking about millions.
Jim:PilotEye has applications for Level 1 automation, which is the category of assistance.
Yemaya:Level two. What about
Jim:Level 2 partial automation?
Yemaya:Yes. Level two. Human pilot still has full responsibility. the AI pilot not flying, provides assistance and forecasting risk in offering mitigation. Again, this could be something where, the, the system not only, identifies that the traffic is there, but it also could say that, there is a bird there and you are on, a direct collision course and it could offer mitigation in, for instance, providing a maneuver. So this could be like a TCAS2. if we, plug this into closed loop with, TCAS2, that, that provides some mitigation. Level 3.
Jim:If I may real quick, yeah, I'm assuming Captain Sullenberger would have, that would have been extraordinarily valuable in this situation where if he could have been notified, I think there were Canadian geese that around this certain direction. I'm not sure what he could have done different. Luka could probably could answer that better than me, but I would assume with that kind of capability in a commercial aircraft, would be a big deal where there's a lot of birds and obviously that had near catastrophic.
Yemaya:Absolutely. Absolutely. And I think that's why, as we're introducing PilotEye, that's why many get it. One operator that we met, he'd been flying helicopters basically his entire life because he learned, to fly from his dad. And he said while he was in, fixed wing training that he almost hit a bird and he, I think he said that he dove and he said, if I had your system during that time, he said, I thought I was going to die. And I could have died. I wish that your system was available to us at that time and, for the same reason, we think that you're right we can't say what could have happened, if Captain Sully had a system like ours, but we would love to believe that our system could have made, a difference in, the impact in the first place.
Luka:Yemaya, can we quickly climb through the ladder and get through level five and then we'll get into the topic of, certifying these systems.
Yemaya:Absolutely. level three, automated, autonomy, or automation, rather. the human pilot, it's either remote or on board, and it's still responsible for the decision making and execution of the system. And the AI, pilot monitoring provides, full guidance and, while the pilot still remains fully responsible. And this could be a system that, identifies a runway and then provides full guidance all the way down, to a safe landing. Level 4 is fully automated. In this case, the human pilot, is remote and supervises all of the AI decisions. And, the AI co pilot, is promoted to the pilot flying while there is a human pilot that is supervising and, can always interject if a wrong decision was made, but in this case, the airframe, the aircraft is self separate in, in the air. And then finally, Level 5 is what we call autonomous, that's the difference between fully automated and autonomous, and that's where there's no human and the, the AI pilot flying, has full authority.
Luka:What
Jim:What would be very cool to know, we're going to talk about certification here in a second, what would be very cool to know is what's the total available market, now, three years from now, five years from now, ten years from now, by level. you don't need to answer it now, but would be interesting is, my guess is four and five are near zero today. and then by level what's the available market for your technology with its application of AI, that would be interesting to know, you don't need to answer it now. Maybe at the end of the podcast or, maybe at another time to see what these opportunities are, in
Yemaya:That's great. Our team is going to go back and definitely take a look at, from that perspective. I love it. That's a great way to slice this as well.
Luka:in preparation of the podcast, we've read your white paper with Intel, I think it was earlier this year, on the future of avionics. and there were some really interesting points that, I'd love to uncover in a bit more detail here. the paper outlines, really two main challenges around the future avionics. One is the software assurance problem for AI and for neural networks, and in this case for Pilot Eye with the use of neural networks and computer vision, And then the second one as these neural networks require really higher levels of computational resources, necessity to use multi core processors in avionics. two really fascinating topics for us. So let's start with the first one or actually you pick, but let's uncover both of these angles in some level of detail.
Yemaya:Yeah. now we're getting into my passion and where I start to nerd out because by training, I am a, Silicon Person. My doctorate is in electrical and computer engineering I did atomic force microscopy looking at, individual, transistors and how, electrons and photons, I'm sorry, electrons and,
Luka:I like that. Infotants.
Yemaya:How they move through individual gates. And so this is really cool. I was at Intel for, what? Seven years before going to Daedalean. And I actually met Daedalean, in my work at Intel in driving, multicore adoption to higher DAL levels in the avionics space. So I love this topic of processing and how to, compute massive amounts of data, on systems that have to meet the size weight and power constraints of gravity. This is, again, on, this, idea of high performance computing, this is where gravity becomes a big challenge. and so I've spent, years trying to support and solve this problem. The challenge with, artificial intelligence is, again, it requires a lot of processing, resources that for certification or systems that needed to be certified, systems that were safety critical systems, it's pretty unprecedented to require this much performance. And, for instance, flight control systems don't require a whole lot. but, when you're working with a lot of data and you're needing to process a lot of data very quickly, particularly that are coming from, image frames, it becomes quite challenging. So this was a problem that Daedalean did not set out to solve when the company was founded in 2016, our co founders were fully intent on solving the software problem. And, that software problem is really around how do we ensure software assurance when it's a machine learned system, and you're running machine learned algorithms. And Daedalian worked with the EASA and published the first guidance or theory on an approach on how to certify machine learning and found that, some of the differences is in, say, Software Assurance, Code Traceability is really huge. Well, when it's a machine learned system, data traceability is just as, or even more important, for those systems. And, we co published with the EASA, a series of papers, CODAN, Concepts of Design Assurance for Neural Networks. And this was the first guidance on how to manage machine learning systems. And we found that really the focus should be on the data management, data verification, ensuring that, your learning process is managed extremely well and, with high assurance and the way you train your models, all these things in, verifying that there's a, the learning process verification. There's the, model implementation. These are all along this process of assuring what we coined with the EASA in this, in these papers, learning assurance.
Luka:Can we talk about learning assurance a little bit more? Because even from the white paper, it left me, wishing for more, especially how you decompose the system level requirements into ultimately data sets, the algorithms, how you create these, offline, independent, annotated data sets. Tell us a little bit more about that particular aspect, how you run this learning assurance and, reach a level that is, by your own judgment, similar to software assurance for running a DO 178 project, for instance.
Yemaya:yeah. we, we said if I were to summarize what CODAN says, it essentially said that we want to ensure that the data that you train on is representative to your operating environment. And everything from there was how do you ensure that happens? And you have to get a ton, collect a ton of data, right? So we have to be basically constantly flying in order to collect, as much data, as we possibly can. You have to ensure that you have some processes and structures around, how you annotate that data. you have to ensure, that you, are managing the training of that, and the implementation, the verification and implementation, very tightly. And this idea of generalization or, ensuring that your data that you train and how you manage that data is representative of the real world. That is probably where the secret sauce is that we did not publish. And, the question, would remain, well, how much data do I need in order to get there? CODAN is really about the process in that way. It's very much so DO 254, DO 178C, right? It's really about the process, right? And operating in a very processed way, making sure that you have traceability, making sure that you are, clearly identifying and, describing your requirements, and that those requirements can be tested, right? And that, you're not using your training data on your testing set or the other way around, right? To make sure, that you're not over training. And so in, the CODAN paper and that approach, is very much guidance.
Luka:What can you tell us about this generalization and this data collection in a way that represents the actual real world environments? We'd love to learn a little bit more about that.
Yemaya:Yeah, I'll give an example. Let's take PilotEye for an example. for PilotEye, we say that we want our system to be able to identify, traffic and, draw a, a box around it. and, in that case, your training data needs to be, a whole lot of data where you are seeing traffic. And where, you want to ensure that your requirements are well constrained. We start out on PilotEye in, daytime VFR. Right. And so that's, more about, well, then when the system goes into operation, we don't have performance guarantees on it at night because it's been designed and the process that we went through in our requirements, in our performance guarantees were around operations and daytime VFR. So that's one example of, how. We walked through, ensuring generalization, again, the secret sauce, and because we are currently, as far as we know, and even what EASA has stated publicly, we're the only company that is, in this process, we had stages of involvement, or SOI audit with the EASA, a few months ago, and that was the first time that had been done. It was a historic, event for us and for the EASA. we're learning along the way of how you actually prove that. that is another one where proving it is, the trade secret. And, that's something that we do plan to publish once we get certified.
Luka:I was just about to ask you that question, so I'm glad you brought it up. as I see it, perhaps there's two kind of sub discussions in this topic. one part of the conversation is addressing the non determinism of the systems. And, perhaps from your perspective, it's unfair to say that it's non deterministic, even though it's not a line by line kind of determinism it still doesn't produce a random result. There is still some kind of, probabilistic, obviously, but in inferring the function that takes the input to the desired output, even though it's not as transparent as line of code, it's still not random. So you can argue that there is some, I won't call it determinism in the DO 178 sense, but, there is some comfort there. But I think the more difficult question, is how do you actually prove that you can achieve safety, and established safety in all of the possible conditions that you can find yourself in. I think that's a much more difficult problem to do, correct? How do you view these two different arguments, and what's the latest regulatory thinking on it?
Yemaya:Yeah. Well, taking for a moment, taking certification out, just in, in software development and software engineering, Determinism is some given input, being operated on by a given operator I have the same output, even by the way, if that output is wrong every single time, but if it is exactly the same every single time, it is by definition a deterministic system, right? And that's what we're trying to get to. We're showing that, if in the way that we treat the data and in achieving generalization. That for given set of inputs, and that set of inputs is well defined in the requirements that, running through our model, we always will get the output, meaning that it'll be identified, say for a pilot eye, as an example. And so that means that we have to be clear and sure, on the where and what conditions it should be, operated. And again, if it's designed for daytime VFR, yes, it turns out that we've actually had some folks that have tested, our system at night and they're like, it works great at night. Well, that is great for us, but still, in getting certified, those performance guarantees are for very specific conditions.
Luka:And so what stands in the way from having some of these frameworks for certifying ML based software be accepted as a means of compliance? What are the main remaining concerns that EASA, FAA, and other regulators around the world have?
Yemaya:The EASA is a bit further along, seemingly at least, with CODAN on how to treat, machine learning systems. I think one challenge and the bigger one that comes to mind right now is just in, having a harmonized approach. And our company is working, pretty closely, in order to drive towards some harmonized approach. Keep in mind that, PilotEye is currently in a process. And it's in a process through the FAA with concurrent validation with the EASA. And we gotta answer some of these questions as we're in the process, and I think we are. this process has been one that, from our perspective, we are we're thrilled, in it. Not saying that, any one of the agencies are, being progressive in any way, that definitely is not the case. however, one thing that we've noticed is that the agencies are really digging in and for instance, through CODAN, the coauthors on the EASA side, they previously had no experience with machine learning and they dug in, they got statistics, they dug into really understanding what is happening here so that they could provide some guidance, that, could support the overall objective of, providing some guidance for acceptable means of compliance. And so I gotta give kudos to both agencies because, they are learning and they are asking us a lot of questions and they are challenging us. And, they are, as we, drive toward this, this development and this effort, we are finding, that everyone wants to ensure that we do the right thing,
Luka:other than harmonization, what are some of the ways that they challenge you? What are some of the questions that they raised?
Yemaya:Yeah, some of the questions, I'll say, Alright, one, one is, our CEO, Luuk, he has published a series of, blogs and blog posts and a video on explainability. And, so this is one area where, our approach and our, thoughts on explainability there could potentially be at least some areas where this, there's, some misalignment, and, so that's one of the areas where we're like, okay, what does that mean? And even if we have, a different approach or, We don't value that approach as much what does that mean for this project? And essentially, what do we have to do in order to prove explainability?
Luka:And in terms of accumulating enough data to represent the real world environment, what's the role of synthetic data in this process? And what are some of the challenges that exist in leveraging synthetic data in simulation? How much is the regulator giving those, quote, credit?
Yemaya:That I can't say for certain, as I'm not that deeply into, the credit. I can say that, we leverage simulated data, for sure. Of course, in aerospace, flying is quite expensive, and so we leverage, simulated data. I can't say how much, but we definitely do in our approach.
Luka:And do you think that startups are at a disadvantage relative to incumbents as it relates to exactly this point of the, the cost associated with obtaining real world data.
Yemaya:Absolutely. Absolutely.
Luka:So what is the role of startups?
Yemaya:I think the role of startups is in, the innovation part. Startups have been phenomenal for driving the innovation that will transform aerospace. I really do believe that. I think startups have some risk, many have some risk of, not remaining super focused on their core IP. and, I see a lot more, vertical integration with, startups than you would for the more traditional aerospace market that it has been moving directly in the opposite direction for the last 10 or 15 years, right? in the broader aerospace market, open systems is everything. The United States DoD, they, at some point, they got sick of, the large air framers, I won't call any names, but the large air framers charging them insane amounts of, money just to change out a chip because For instance, it was something that it was an ASIC and it wasn't commercially available. It wasn't commercial off the shelf. So this, idea of vertical integration, there are parts of it that can be, a distraction for some startups that are, developing airframes. And I think that, your time to market is really important. you don't get credit for efficiency, you get credit for delivery focusing so much on your core IP and making sure that you're better than anyone else in the entire world on your core IP and outsourcing basically everything else when it is feasible and when it's viable, and leveraging the existing ecosystem. I believe those are the folks that are going to win.
Jim:As you were doing your certification with EASA, I'm assuming this is certification level C. is it, essentially level 1 through level 3? Okay.
Yemaya:This is, well, two different things. DAL C, that's Design Assurance Level. Pilot Eye is, we're taking that to DAL C. That is separate from, the levels of automation, the PilotEye, we'd consider that to be a level one, assistance, pilot assistance system.
Jim:But none of their certification so far is DAL A. You haven't, DAL B or to DAL A? Okay. It's all DAL C.
Yemaya:Oh gosh, yeah, Yeah, just so that we're reminded, there is currently, I believe there's currently only one computer, that is certified to DAL A, that is, on a multi core processor. That is running a CPU that is, I believe, already end of lifed, or close to end of lifed. and... A GPU that has already been end of lifed, this takes a long time, right? And so add AI to it and I think that, our first goal in delivering product and delivering, value has been, we just want to prove that you can certify AI. And once we prove that, right, then we can drive toward, higher levels, but keep in mind as well that you don't start with, I want to build a DAL A system or I want to build a DAL B system or DAL C system. You start with the intended function. And, you take your intended function, and then you break that into sub functions, and you, apply a functional safety or, hazard assessment, against those, you assign a hazard classification from level A through E, with A being the highest safety level, safety criticality, that's like a flight control system. With a level E being no, impact to safety. That is the toilet or the, in flight entertainment system. and only when you have a hazard classification, do you start designing your architecture because that tells you how much, mitigation that you need to apply, to any potential, hazards or, safety hazards. And those, mitigations can include redundancy, dissimilarity, for any commercial aircraft the flight control system is not only redundant but dissimilar. And there are flight control computers that are controlling individual flaps, or flight control surfaces, but those are copy exact two to three times, sometimes even four times. And then separately, those flight control computers are designed by two different companies, two different teams, and in most cases, even two different silicon, such that they want to ensure that there is no opportunity for, common mode errors when designing the software. For instance, if I, I have one piece of software that, leads to a fault, that, that does not cascade to every single flight control computer, on my airframe.
Luka:Yemaya, let's talk about multi core processors, MCPs. You mentioned that there's only one DAL A certified MCP, and it's using end of lifed CPUs and GPUs. We talked briefly about why AI applications need MCPs for the higher... compute requirements of, running, AI based algorithms on it. But why are MCPs so difficult to certify?
Yemaya:Yeah, MCPs are great. In the same way that MCPs are being used, in commercial electronics, for the higher performance, for, the lower power consumption, for the improved efficiency, scalability, being able to execute multiple workloads concurrently, low swap, all those things, are the reason for consumer electronics. Same thing in the Airframe. The issue, though, is that when you need to be certified, the software that's running on the, processor can no longer control all of the behavior of the silicon. So, for instance, in a single core processor, the software, and by software I mean the, the real time operating system, folks like, WindRiver, Lynx, Greenhills, they build this software, in, in that case, you're not competing for resources, you have only one core, you that is doing all of the computing, all of the processing and heavy lifting. And that one core is not competing, with another core for resources, more specifically memory, memory and cache, right? And so when you add additional cores, though, you lose the ability for the software to, control, all of the behavior of the processor. And so now, Core 1 and Core 2, or Core 1, Core 2, Core 3, Core 4, they're all competing for registers. They're all competing for memory, and to put an instruction, into memory, right? And what they generally do today is you start with partitions, and they partition based on the DAL level. And, the overall goal is to achieve, or bound, the worst case execution time. So that means that, let's say, I have, a system that is doing, some navigation, but it is also running, some, say, flight controls. pilot Pull Side Stick, that is a DAL A process, and there should be nothing that is possible that could interrupt that. There should be nothing, no way possible, that instruction, can be kicked off of memory because something else has come up. And, that other thing is now the higher priority. This happens, in consumer electronics, they're actually designed to do these things because, for performance, right? And so I, if I'm working on, Microsoft Word and I switched to another application and now I'm going to start streaming and I go back to my Microsoft Word, right? when I'm clicking back and forth and I'm telling it to do stuff and, play here, but also I want to go back and I want to start typing, for performance requirements, there are times where, for instance, instructions can be kicked out or they can be de prioritized and they're designed to do that. that's how you're able to get the gains that you are from, multi core processors. But, there should never be a case where a DAL C, application such as, displaying, traffic is more important than being able to control the airframe. And, this is why it's... It can be difficult, to certify MCPs because it comes down to a software issue and the software, not having, in the MCPs. The consumer processors not being designed with the tools for the software to monitor the processes, to control the processes fully, and ultimately to bound the worst case execution time. And, in these designs, we turn out to actually, I just say throttle the performance of the MCPs in order to make these guarantees for as much as we can on, developing the software that is going to run on, the processors.
Luka:And so, to what extent is certifying MCPs a purely technical problem of making sure that all of these interference channels and this fight for resources is managed? As opposed to the challenge of just not having visibility into the processor architecture because one of the things that you brought up in the white paper is that, silicon manufacturers are not very keen in sharing that level of detail that you would require in order to build this, resource sharing or mitigation that you mentioned. So, Which is it? What's the main problem? And in the case of, Intel through, the Airworthiness Evidence Package, sharing these... Certification Artifacts, does that solve the problem or is there still a problem remaining?
Yemaya:Yeah, This is, it's such a great question. So the airworthiness, evidence package at Intel, that is my claim to fame, I, co architected, safety critical avionics solutions and products for Intel, that product line is my baby and, where I really started in, safety applications, in aerospace. And the issue for the developers of these systems, they have to ultimately be able to predict every possible, failure condition that can happen, and that means that they need to be able to predict the behavior, every possible behavior of their system, right? Their ability to do that is limited to how much information they know about what the processor does and why it does what it does. And the Airworthiness Evidence Package. was about providing, that transparency to the developers, to the embedded, OEMs who are developing these systems. We, went through an analysis with them, and we wanted to understand, what is it, when you say that you want us to support you, OEMs, of the world who want to design in Intel. What is it that you actually need? What do you want? what information are you looking for? And initially when we got that information from them, we realized that there was a good amount of it that was trade secret. to the point that. it wasn't even available to just broadly to the company. And the second issue that occurred was that, the aerospace volumes are just not there. to get a company that is producing millions of processors per day for, consumer electronics orders and orders of magnitude, more volume to get them to operate outside of the process and provide transparency. initially there's, there was no real opportunity there, but what changed that was, it started with the autonomous driving, And a lot of what was necessary was needed for that as well. And Autonomous Driving immediately, they saw that, these are going to be volumes upon volumes of essentially then we also had the opportunity to be part of driving data centers, and there was a big opportunity. And so the aerospace teams, myself, in leading these efforts, we took advantage of what was already happening in order to support the automotive industry, and we conducted a gap analysis between the DO 254 with the, the 26262, which is the, safety guidance, on the automotive side. And for the data, there was data that already, existed, but we generally would not provide. This included, single event upset data, which we would never provide this data because in, in many ways, a knowledgeable, company with this information could essentially reverse engineer, the, architectures and get trade secrets, if they had that information. And so we provided that. We provided, then some, functional safety, failure modes, and errors diagnostic analyses, so we provided failure rate, we ran it through tools, in order to identify, some very critical, behavior of the silicon, such that then when it, was integrated into or would be integrated into a, system that the developer had enough information, that they could provide mitigations. It doesn't mean that you necessarily control it. It could mean that, okay, well, because I don't know when it's going to do this, or, this fault will happen in these very specific conditions. Now I have that information. Maybe that means that I need to provide redundancy and I need to run a separate, processor alongside it and then do some kind of checks, or maybe I need some kind of monitor of, another, processor, such as, say, like an FPGA that is monitoring the silicon and can do something when, this failure condition occurs.
Luka:do you think that there is a, path to a generalized approach to certifying MCPs as opposed to having to go through, a lot of, detailed analysis every time that there's a new processor on the market?
Yemaya:I, I think that we're probably about as generalized as we can get, the guidance is meant to, support, what is currently available. It's, CAS 32A, and I think it's the 193? Now, CAS 32A, I believe, is 193. And, so I think we're possibly about as generalized. When there's new silicon, there needs to be detailed analyses and particularly when silicon is meant and designed for a non safety application.
Peter:So if you take one of these MCPs this certification process what's the expectation in terms of how many years it will remain technologically viable in the market? how much time do we get out of these things before either it becomes obsolete or before it's no longer available in the supply chain and you just have that inventory and that's it? What does that look like?
Yemaya:Yeah, these things go into service for 20 and 30 years. when you have an OEM, an air framer who is aligned on the silicon, you have to be ready to support them for decades. because the cost that are required in order to, provide assurance for these systems are so great. I'm only saying, right now we're talking about just the design, right? Software is its own monster. there was one case where I met with an OEM, a developer, and they said that, they had converted from Intel, by then they had converted from Intel into Power Architecture. By the time I was meeting with them, they said that it was like a decade ago. They said that still they were spending$1 million per year on the architecture, change. And this was in software investment. So it is great, the investment in, getting these things certified. And so they're expected to run, basically forever, essentially. and by the way, this is exactly why when there is a system that goes to market, when it goes to market, there are many stories that folks, particularly on the military side, they can say. I think the worst example was, I had a conversation with an officer. in a military agency that said, the day after we went into service of this airframe, the following day, we received a obsolescence, notice on the silicon this happens often. It's normal.
Peter:Wow. That's such a long timeline for these products. It's totally different than... other industries, and obviously it has all of these downstream implications for, how It's employed in higher level systems, obviously, which is fascinating.
Yemaya:Absolutely. Absolutely. And so this is why, we say, well, that we have that one system that's available. And I think it was launched last year, I believe. And, a year later is, the end of life.
Luka:Yemaya, would you... encourage innovators to develop multi core computers, and taking them through certification, ground up, or are you a proponent of taking whatever is best of breed in the commercial world and somehow running it through the cert process?
Yemaya:Yeah, it's not really possible to take, a consumer electronic device, and get the higher DALs, it starts, let me take that back, you have to have an insane amount of information that most consumer electronics companies don't, that information doesn't even exist because they don't test for certain things. and so it is very difficult to start with, a consumer electronics device and meet high DALs. And certification starts from day one and from the ground up and there are many companies, by the way, that are developing, rugged, certifiable computers. There's an entire ecosystem that has been created in the last 10 to 15 years that's fully dedicated to developing these systems and I say that's one area where you should just work with the existing ecosystem.
Luka:How do you think that autonomy and certification of AI and MCPs change the existing value chain in avionics and how value is distributed. How do you think that the Avionics value chain will be disrupted?
Yemaya:This is a great question. We're seeing a good amount of this across the tech industry as a whole. And, in this market today, it's the avionics OEMs and the air framers who are relied upon to drive innovation, but they are relatively disincentivized to do it. Why? Because the investment for, commercializing, a certified product is so high. And then once you make that investment, you have to then focus on, maximizing your profit. And that means that your assembly lines have to be running nonstop, right? And so when you need to, I guess optimize for, filling your assembly lines, you're disincentivized to innovate because it requires more investment, and it eats into your ability to be profitable, in selling your systems, but what I'm seeing lately is that, this interesting shift is that tomorrow's operators and end users, they're starting to drive some demand for capabilities. They're starting to drive demand for, say, our system, PilotEye. We're finding that it's the operators that are saying, we want this, and we want you to deliver this. And, we're working along the value chain in a way that we didn't expect to be operating along the value chain and traditionally they would apply pressure on the air framer in the avionics OEM in order to integrate and we're seeing some very interesting cases where, they're saying, no, I'm just going to work with you directly. I think one example could be, let's see, Air Methods with Skyrise, right? And in, in that way, I mean that, this is not something, a deal that's including Garmin. It's not a deal that's including the air framer. This is a company that is, delivering directly to, an operator. I think that is relatively new. now how we see this shifting the value as well is, I think that in driving in this direction, this trend, it is potentially going to lead to the hardware, essentially being democratized in a way that the value becomes the software versus the hardware. One example is that there was a time where in operations, just having a camera was huge you had all this additional situational. awareness with a specific kind of camera. Now, once you apply artificial intelligence to it, suddenly the operator's like, No, it's the AI. It's the insights that are delivered from the AI that's where all the value is. And, I think that this is going to be an interesting next, decade in seeing how the traditional avionics OEMs and airframers react to this. another example in consumer electronics is, everyone's, PC, at least ones that have Intel people have a sticker that says Intel inside. No one knows why that even matters, right? So people know Intel because they knew the sticker that was on their computer. but where they saw the value was in Microsoft, right? they see the value in Microsoft. They see the value in Apple, which is, mostly, on the OS and the applications that are built on top of the OS, but not realizing that there are certain functions, For instance, remember when there was a time where you would be typing up a paper on Microsoft Word and your computer goes out and you are screwed. You've lost everything if you did not do a save, right? It was a function in the silicon that enabled the software, to do continuous saves, move, a current instance into, non volatile memory and keep that such that when you turn your computer back on, you can go and get your document. Well, that, that was the Intel inside that, that enabled that. and I think that we're driving in a direction that might look the same way on, the, avionics side and then aerospace side.
Jim:Hey, my question, you can answer or not answer any of these questions, but is 80 percent of your revenue today coming from GA
Yemaya:Yes.
Jim:And two three, four years from now, how will that change?
Yemaya:I think it'll still be mostly GA.
Jim:And 10 years from now, what percentage of revenue do you think will be from advanced air mobility?
Yemaya:Oh, 10 years from now, Advanced Air Mobility? it will be larger than the first five years? Haha, it, that's a good question. again, I think, I do believe this goes back to our first question, that, it, we, there will be revenue that is because of advanced air mobility. In fact, I think that if we are successful, it'll be a large amount and, by successful, I mean, that we are directly addressing the cost, we're directly addressing the, resource efficiency and able, to introduce systems that, are commercially viable because there is some level of automation.
Jim:In order for us to get to level 4 and 5 and DAL A and B, what's the greatest limiting factor? Is it your ability to be able to create the capabilities to be at level 4 or 5? Or are there other factors that you think would be limitations?
Yemaya:I... I don't know that it's necessarily a technological problem. I do think that it's a regulatory issue. There's also parts of it that is, even a perception problem. so many people think AI and they think Terminator. And, we're like, you can also use AI to do boring stuff, just identify non cooperative traffic, right? And so... It's the responsibility of folks like Daedalean, and we're taking this on directly. It's our responsibility to, not, integrate AI and applications that just don't need it, right? And for PilotEye... If it's cooperative traffic, it outputs ADS B. That's great, right? But we provide additional, value in using the AI just for non cooperative traffic. use the technology very wisely and where things are working, take advantage of that. We do believe that, AI enhanced autonomy is the best chance of operating, in environments that are not so constrained that they might completely eat away your economic viability in a way that your operational constraints are so great that you can't scale or, you can only operate for these very specific con ops that turn out to, oh, by the way, they are, low margin or low volume, or they don't scale themselves. Right. So we try to really pay attention to that. And, we take the approach of where there is a technology that exists and it works, we want to do that. And we start with how can AI augment that and support that to ensure that, it enables, more freedom in the way that you can operate.
Jim:5, 10, 15 years from now, paint a picture, if you could briefly, just paint a picture of the level 4, level 5 automation, what's a high probability future look like?
Yemaya:Yeah, I think that, there will be cases of level four automation in very constrained operating environments. in 10 years, I think that there will be, less constraints, possibly still mostly fixed route, automation, in some specific con ops, and potentially there might be one or two con ops that, are fully autonomous, again, in very constrained, operating environment. 15 years, I think that's closer where we start seeing some real scale and commercial viability.
Jim:So you're saying, at the same time, that's probably the future of autonomy for advanced air mobility as well, and you're saying that's the viability of advanced air mobility on the same timeline, given your initial
Yemaya:comments. Right. the tech needs to be proven out and, our company, we're proving out the tech in today's airspace in GA, we don't take the approach that automation and autonomy is an all or nothing, kind of proposition. We say that, we can address this by, leveraging the technology in today's airspace in, limited ways, augmenting the, human pilot in, some ways that then by the time, Urban Air Mobility is ready to scale the technology has also been proven out and has had years of certification credit in order to be proven out and then integrated.
Jim:Can Daedalean meet its financial objectives at just level one and two levels of automation in the next five years?
Yemaya:I believe so. Yeah, I think that we have options. The great thing about what we're doing is not only are we building systems, pilotize a system that gets sold to operators, but we have built a lot of IP in the meanwhile. So the white paper was about proposing an architecture, right? In the meanwhile, we will have the most advanced, and highest performing certified computer that is available. And there is a commercial need for that, right? We have IP, that we are developing all so that we can just deliver, the, machine learn systems. And so I think that provides us with the, ability to leverage and, and take advantage a lot of today's market across segments, in fact,
Jim:Yemaya, what final message would you like to give our audience? This has been a great discussion.
Yemaya:Final thoughts. Automation, autonomy. is going to, provide outcomes that will eventually be immense, and the folks that will, benefit the most are operators and the end users who are, the customers to the operators. Automation and Autonomy will also transform aerospace as a whole across all segments of aerospace. and Daedalean will continue to lead the way in, ensuring that we can realize this world that, produces and, makes possible, automation, autonomy, and we're doing that with artificial intelligence, and, we're really leading the world in how to, implement artificial intelligence.
Jim:Amazing, Yemaya. Thank you so much for joining us.
Luka:Yes, thank you. This was a phenomenal conversation. Really enjoyed it a lot. Thank you so much.
Yemaya:Thank you for having me.